Manufacturing analytics is a powerful data-driven methodology that can help you uncover the cost drivers in your operations. In one of our previous blogs, you can read more about how to uncover these cost drivers with the help of manufacturing analytics. However, practice tells us that a staggering 87% of analytics projects in the industry fail to deliver the expected value to the business. They end up in pilot purgatory.
One of the primary reasons manufacturing analytics projects fail is the misconception that simply investing money or implementing technology will guarantee success. This approach is not sufficient to achieve the desired results. The other key player is data, which is a double-edged sword – it’s what makes all these analytics and capabilities possible, but most organizations are highly siloed, plagued by insufficient collaboration and ineffective communication. A structured approach to your analytics transformation can help mitigate this risk (figure 1).
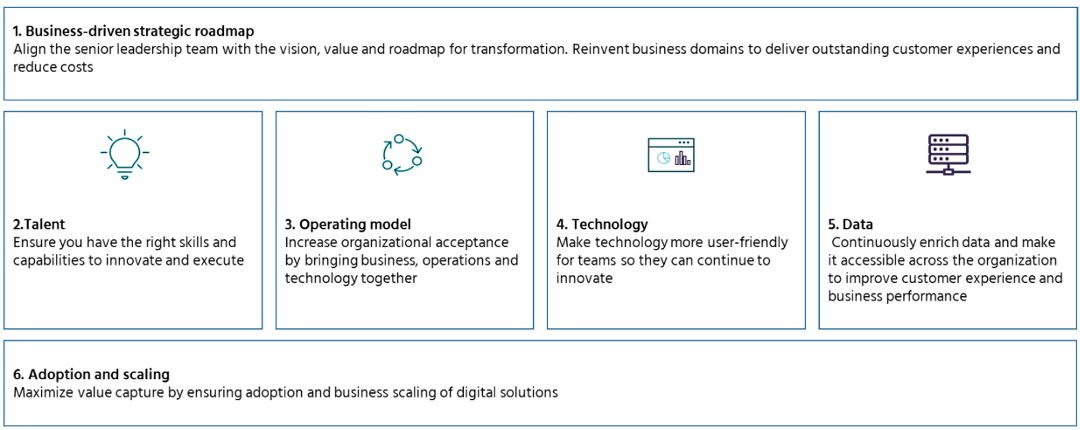